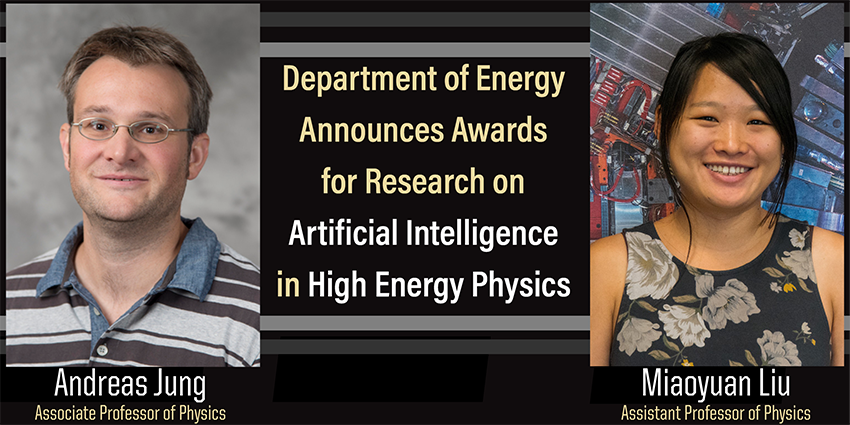
Congratulations to our distinguished CMS researchers who have been awarded a grant for their research by the US Department of Energy [DOE announcement]!
The grant is for a variety of projects in high energy physics, and focuses on the use of artificial intelligence and machine learning - key areas that could access otherwise inaccessible areas in top quark physics for further research.
We caught up with two of our winners, Drs Jung and Liu.
Well done on receiving the grant - it is high praise indeed for all your work. Could you start by telling us a little about the research you’re involved in?
Miaoyuan Liu: I've worked on a broad range of physics topics including studying the electroweak forces with multiple bosons and directly searching for new physics predicted by symmetry structure beyond the Standard Model. I am a believer that advancement in instrumentation and experimental techniques will lead our way to discoveries. Machine learning and advanced computing show great promise in helping us with tackling challenges in studying the rare and elusive in the enormous data at the extreme rate produced at the LHC.
I am also contributing to the CMS outer tracker upgrade for the High Luminosity (HL) phase of the LHC Runs [upcoming after 2029, read more here], which will capture and measure new physics with a dataset ten times larger than before.
Andreas Jung: My research focus is on top quark physics and centres on the analysis of proton-proton collisions recorded with the CMS detector at the LHC. My group is deeply involved in the upgrade of the CMS detector for the High-Luminosity phase of the LHC. In particular, I focus on top quark properties by means of precision differential measurements of top quark production that have the ability to shed light on the inner workings of the electroweak symmetry breaking and what stabilised the electroweak vacuum. New physics is expected to show up in this realm and deviations from the Standard Model of top quark physics can hint at beyond the Standard Model contributions.
How does your work fit into the wider research questions in physics?
Andreas Jung: The field of top quark physics is at a turning point transitioning from analysing millions to, by the time of the high-luminosity phase of the LHC, billions of top quarks. The vast data sample will finally even allow us to understand and shed light on elusive quantum mechanical phenomena, such as the quantum entanglement of top quarks and the violation of Bell’s inequality. Both are fundamental effects in the realm of quantum mechanics that can finally be observed and have been at the heart of the 2022 Nobel Prize award in Physics on Quantum Information Science.
Miaoyuan Liu: The current and future LHC runs deliver data at unprecedented extreme rates that pose challenges in identifying, collecting, and scrutinising new physics signals in collisions. The current CMS online system handles petabytes per second of data produced by proton beams colliding at 40MHZ and the offline distributed computing system processes a few petabytes of data per year. This presents both challenges and opportunities in applying Machine learning (ML) in each tier of these systems.
When you think that just one petabyte has been equated to 500 billion pages of standard printed text, and two petabytes are enough to contain all the US academic research libraries, it is an enormous amount of data!
Let’s come back to the grant itself - could you explain how this allows you to expand on your research?
Andreas Jung: The grant is to develop cutting-edge artificial intelligence and machine learning (ML) techniques that go beyond what has been done for many years in high-energy physics. These techniques will become a vital part of a ‘toolbox’ to harvest the full information content of data collected at the LHC. We employ earlier work on interpretable and trustworthy geometric learning techniques to overcome shortcomings in current techniques that are limiting our ability to understand the production of the heaviest elementary particles, the top quark. The US Department of Energy “AI for HEP” (Artificial Intelligence for High Energy Physics) seed grant provides the means to significantly improve the precision with which top quarks can be reconstructed and measured in proton-proton collisions. Our work will open the door to potential new discoveries and improve our understanding of the Standard Model of particle physics.
Miaoyuan Liu: My current work focuses on Nanosecond ultra-low latency (aka super ultra fast, 1 out 10 million of a second) Geometric Deep Learning (GDL) for real-time systems such as our CMS trigger. To make sure we can trust them to filter new physics in an online system, I developed methods to understand what information the GDL is using for the decision making. These techniques can be adapted to better reconstruct top quarks and study them more in depth. We will also leverage the efficient processing of large data sets with heterogeneous computing resources, to see if we can leverage the df for the most precise top quark measurements, a topic deemed 'impossible' at the LHC!
Thank you both for your time, and we look forward to the contribution this brings to the wider experiment here at CMS.
Disclaimer: The views expressed in CMS blogs are personal views of the author and do not necessarily represent official views of the CMS collaboration.