Kevin Pedro is a physicist at CMS who recently won an Early Career Research Program grant from the US Department of Energy for his research in Dark Matter. The program supports over 80 early career researchers for five years at U.S. academic institutions, DOE national laboratories, and US Office of Science user facilities.
Here we interview Kevin to find out a little more about himself and his research:
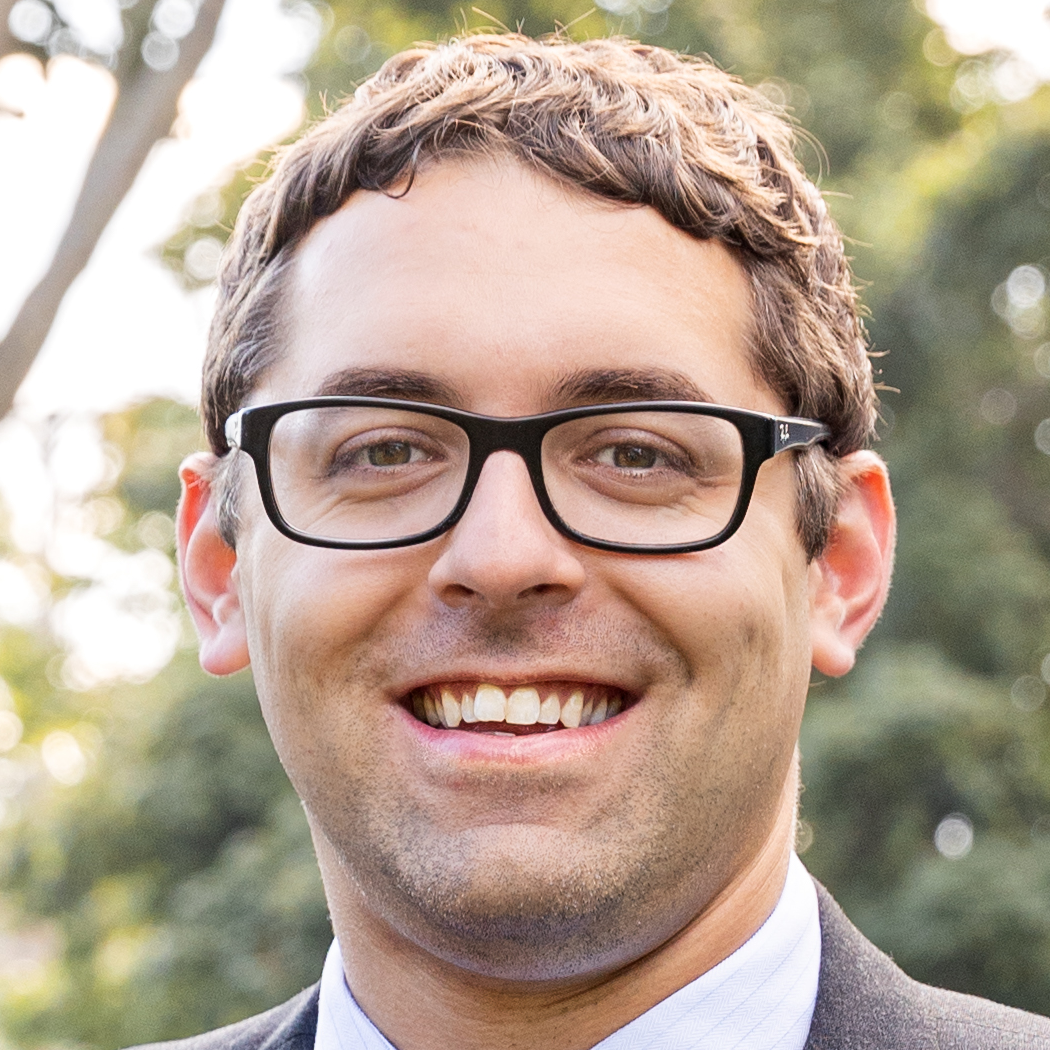
Hi Kevin! First of all, congratulations! How are you feeling now that you’ve won the grant?
I am eager to make progress on all of these interconnected projects! I find it very fulfilling to have the opportunity to lead this research that I really believe in.
Tell us a little more about the research you are involved in: what is it all about and why is it important?
The nature of dark matter is one of the great unanswered questions in physics today. We know that it exists—galaxy cluster collisions and the cosmic microwave background spectrum are the most conclusive pieces of evidence, to me. But we don't know what kind of particle it is. We've made many attempts to probe dark matter more directly: by producing it at colliders, looking for any interactions with ordinary matter, or observing it annihilating with itself. So far, we have no clear signs. We've excluded a lot of models, but the remaining possibilities are still vast.
The idea of a complex dark sector caught my attention in 2015, when Professor Mariangela Lisanti presented the idea of "semivisible jets" at the LHC Physics Center at Fermilab. These models postulate that dark matter isn't just one elementary particle, but rather the result of a whole new sector of particles and forces, similar to the quarks, gluons, leptons, etc. that make up ordinary matter. The dark matter would then be a composite particle, like the protons and neutrons that make up most ordinary matter. Most of these models can only be accessed at colliders, and the signatures are very different from other dark matter searches. That means there might already be dark matter events in the LHC data, and we just have to look for them differently. My primary focus is the semivisible jet signature that I mentioned, where there are invisible dark matter particles mixed into something that otherwise looks, at first glance, like a hadronic jet from the standard model. I collaborate with a great team of professors, students, and postdocs at Fermilab and both US and European universities, and we use various machine learning approaches to distinguish between semivisible and standard model jets by looking at their substructure.
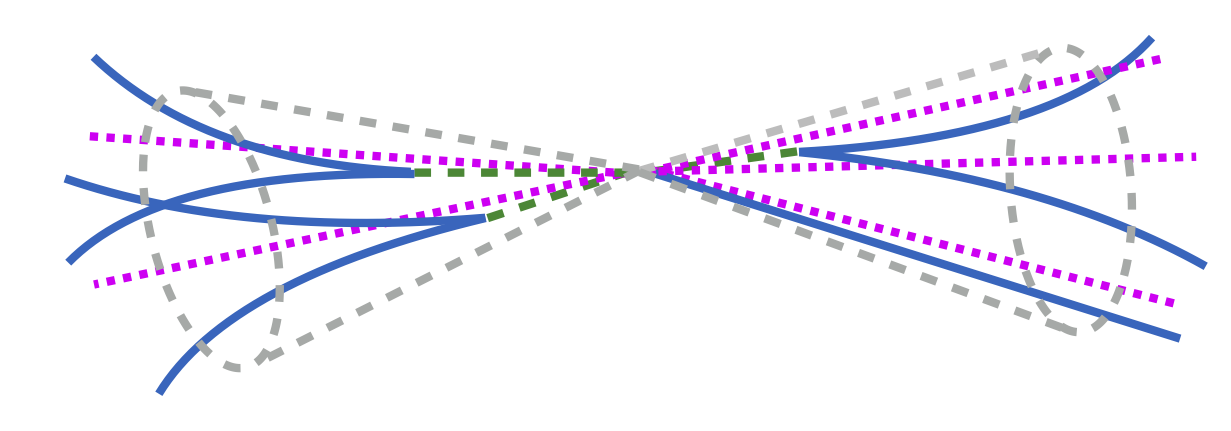
These "dark sector" models have quite a few unknown parameters, so we have to explore many different possibilities. Also, the main background is just standard model multijet events, which are produced very plentifully at the LHC. That means we need to simulate a lot of events to make predictions and design an effective strategy. Simulation is very computationally intensive, so we're trying to employ machine learning here as well, to speed it up while retaining high accuracy. I am pursuing diffusion models, which are also used heavily in industry for image generation and other similar tasks, because they produce the highest-quality output. Our plan, currently in progress, is to deploy machine learning-based simulation on GPUs, so we can run the algorithms as fast as possible. This is key to facilitate these broad searches, especially in the upcoming High Luminosity LHC era, where we'll have ten times as much data, and we'll need a corresponding amount of simulation.
How will the grant allow you to progress your research?
The grant will support me and a team of expert personnel, both physics and artificial intelligence researchers, to develop new techniques for both the semivisible jet searches and the machine learning-based simulation. We want to be sensitive to as many possibilities as we can, so a major part of the project is to make the results more model-independent. To do this, we will primarily rely on anomaly detection. At the trigger level, this lets us record unusual events—like semivisible jets—that existing triggers, based on more conventional signatures, might miss. In the final analysis, anomaly detection can reject standard model backgrounds while minimizing any bias or preference for one signal model over another. These techniques are usually called "unsupervised learning", and they are closely related to "generative learning" techniques like diffusion models for simulation. We are also exploring interpretable learning to get the best sensitivity possible without sacrificing our understanding of the physical properties of any signal that we find. The grant also highlights the importance and promise of this research.
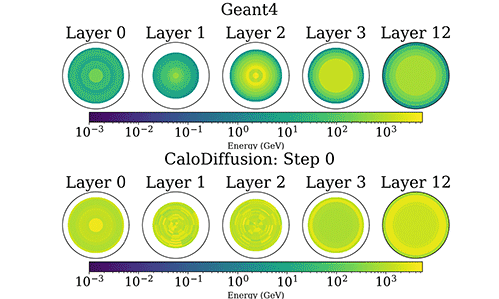
And finally, do you have any words of advice or words of motivation for similar researchers?
I have two pieces of advice:
- Persistence matters! It took me three tries to get this grant, and there were plenty of other grants before this that I didn't get. Keep refining your ideas, as well as how you present them, and don't get too discouraged.
- Run your ideas and proposals by people outside of your group, institute, and even field. The importance of the proposed research should be clear to any reviewer, whether or not they have the same background as you.
Thank you and best of luck!
For further information:
DoE Early Career Research Program: https://science.osti.gov/early-career
Kevin’s work on semivisible jets:
First CMS search: JHEP 06 (2022) 156, arXiv:2112.11125
First demonstration of anomaly detection: JHEP 02 (2022) 74, arXiv:2112.02864
More sophisticated anomaly detection: CMS PAS MLG-24-002
First use of interpretable machine learning: SciPost Phys. Core 6 (2023) 067, arXiv:2303.16253
Summary of CMS dark matter searches, including complex dark sectors: Phys. Rept. (in press), arXiv:2405.13778
Kevin’s existing work on diffusion models for simulation:
CaloDiffusion model (used for the animation above): Phys. Rev. D 108 (2023) 072014, arXiv:2308.03876
Community challenge comparing CaloDiffusion and other models: arXiv:2410.21611
Are you at CMS? Do you have a similar blog you'd like to write? Let CMS Communications know!